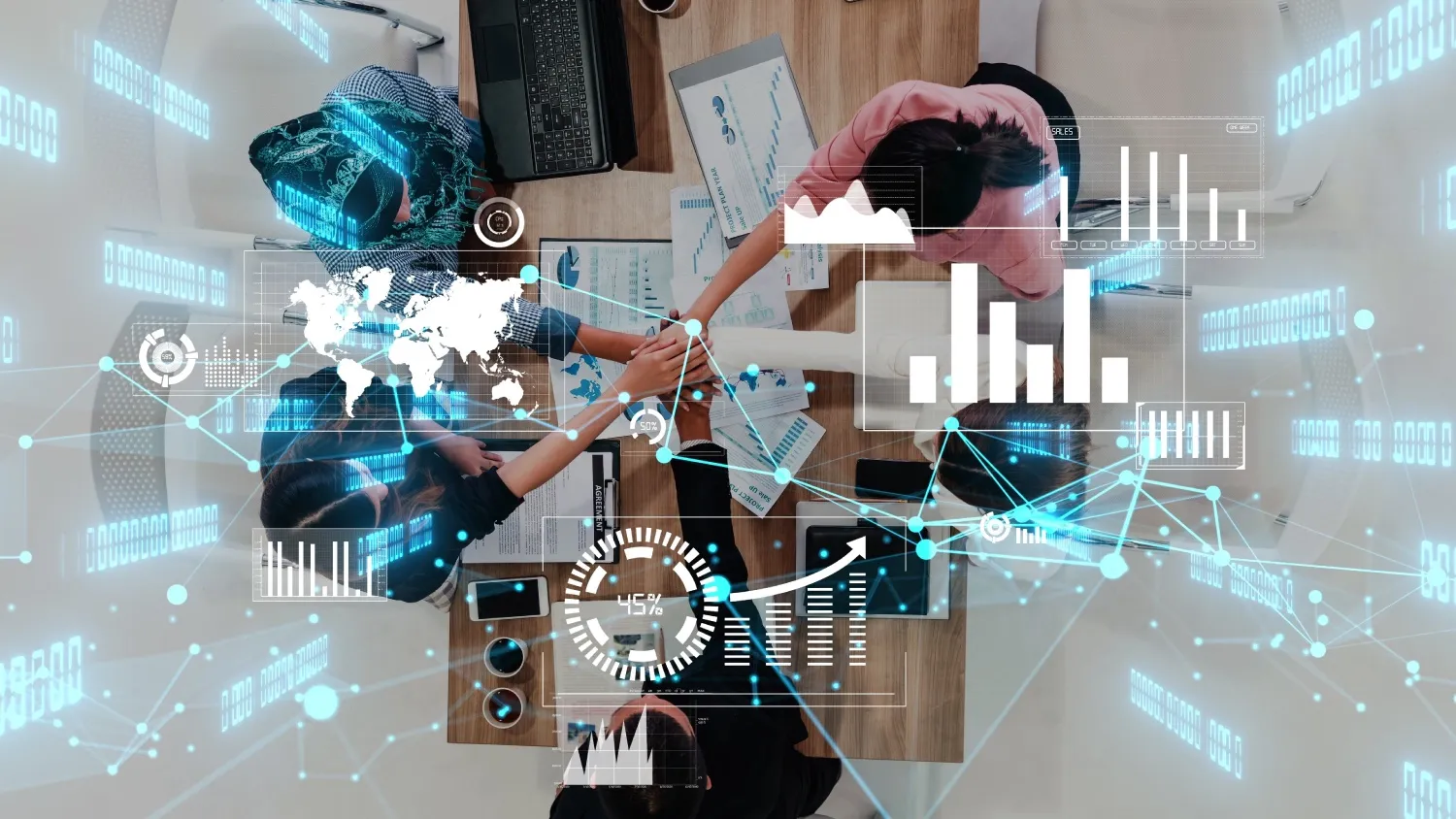
In the rapidly evolving landscape of digital product engineering, the role of data engineering has become more critical than ever. As organizations strive to create more intelligent and responsive digital products, the integration of Generative AI (Gen AI) is poised to revolutionize the field. This blog explores how data engineering is set to transform digital product engineering, driven by the capabilities of Gen AI.
The Evolution of Data Engineering
Data engineering has always been the backbone of digital transformation. It involves the design, construction, and maintenance of systems and architectures that allow data to be collected, stored, processed, and analyzed efficiently. Over the years, data engineering has evolved from simple data processing pipelines to sophisticated, scalable architectures capable of handling massive amounts of data in real-time.
The Rise of Gen AI
Generative AI refers to a class of artificial intelligence models that can generate new content, including text, images, audio, and even code, based on the patterns learned from existing data. Unlike traditional AI, which focuses on prediction and classification, Gen AI can create entirely new data sets, making it a powerful tool for innovation.
How Gen AI Enhances Data Engineering
- Automated Data Integration and Cleaning
- Data integration and cleaning are among the most time-consuming tasks in data engineering. Gen AI can automate these processes by learning from historical data patterns to identify inconsistencies, errors, and gaps. This not only speeds up the data preparation process but also improves the quality of the data used in digital products.
- Advanced Data Modeling
- Gen AI can enhance data modeling by automatically generating complex models that are difficult for humans to conceptualize. By analyzing vast amounts of data, Gen AI can identify intricate relationships and patterns that can be used to build more accurate and robust predictive models.
- Real-Time Data Processing
- The demand for real-time data processing in digital products is increasing. Gen AI can facilitate this by optimizing data pipelines to handle streaming data efficiently. This ensures that digital products can respond to user interactions and environmental changes instantaneously, enhancing user experience.
- Enhanced Data Security
- Data security is a critical concern in digital product engineering. Gen AI can help detect and mitigate security threats by analyzing data access patterns and identifying anomalies. This proactive approach to data security ensures that sensitive information is protected, maintaining user trust.
- Scalability and Flexibility
- As digital products grow, the underlying data infrastructure must scale accordingly. Gen AI can dynamically adjust data architectures to accommodate increasing data volumes and changing requirements. This flexibility allows organizations to innovate without being constrained by their data infrastructure.
Real-World Applications of Gen AI in Data Engineering
- Personalized User Experiences
- By leveraging Gen AI, data engineers can create highly personalized user experiences. For example, streaming services can use Gen AI to generate personalized content recommendations based on users’ viewing histories and preferences.
- Predictive Maintenance
- In industries like manufacturing, Gen AI can analyze data from sensors and equipment to predict maintenance needs. This helps in reducing downtime and improving operational efficiency.
- Smart Cities
- Gen AI can process data from various sources, such as traffic sensors, weather stations, and social media, to optimize city operations. This includes improving traffic management, enhancing public safety, and optimizing energy consumption.
Challenges and Considerations
While the future of data engineering with Gen AI is promising, there are several challenges and considerations to keep in mind:
- Data Privacy
- Ensuring data privacy while leveraging Gen AI is crucial. Organizations must implement robust data governance frameworks to protect sensitive information.
- Ethical AI
- The use of Gen AI raises ethical concerns, such as bias in AI-generated content and decision-making. It is essential to ensure that AI systems are transparent and fair.
- Skill Gap
- The integration of Gen AI in data engineering requires a skilled workforce. Organizations need to invest in training and upskilling their data engineers to leverage Gen AI effectively.
Exploring the Advantages and Obstacles of Automating Data Engineering Tasks with Gen AI
The field of data engineering is experiencing a significant transformation, driven by the advent of Generative AI (Gen AI). By automating various data engineering tasks, Gen AI promises to enhance efficiency, accuracy, and innovation. However, this transition also comes with its own set of challenges. This blog delves into the advantages and obstacles of automating data engineering tasks with Gen AI.
Advantages of Automating Data Engineering with Gen AI
- Increased Efficiency and Productivity
- Automated Data Cleaning: One of the most labor-intensive tasks in data engineering is data cleaning. Gen AI can automatically detect and correct inconsistencies, missing values, and errors, significantly reducing the time and effort required for data preparation.
- Streamlined Data Integration: Integrating data from various sources can be complex and time-consuming. Gen AI can automate this process by understanding and mapping different data formats and schemas, ensuring seamless data integration.
- Enhanced Data Quality
- Consistent Data Processing: Gen AI ensures that data processing tasks are performed consistently, reducing human errors. This leads to higher data quality, which is crucial for accurate analytics and decision-making.
- Real-Time Data Validation: Gen AI can perform real-time data validation, identifying anomalies and inconsistencies as data is ingested. This proactive approach helps maintain data integrity.
- Advanced Data Modeling
- Automated Feature Engineering: Feature engineering is a critical step in data modeling. Gen AI can automatically generate relevant features from raw data, improving the performance of machine learning models.
- Complex Model Generation: Gen AI can create sophisticated data models that capture intricate relationships within the data. These models can provide deeper insights and more accurate predictions.
- Scalability and Flexibility
- Dynamic Resource Allocation: Gen AI can dynamically adjust data engineering pipelines based on the workload, ensuring optimal use of resources. This scalability is essential for handling large volumes of data in real-time.
- Adaptive Pipelines: As data sources and requirements evolve, Gen AI can adapt data pipelines to accommodate new data types and processing needs, providing flexibility and agility.
- Cost Reduction
- Reduced Labor Costs: By automating repetitive and time-consuming tasks, Gen AI reduces the need for extensive manual labor, leading to significant cost savings.
- Optimized Resource Utilization: Efficient data processing and resource allocation reduce operational costs, making data engineering more cost-effective.
Obstacles to Automating Data Engineering with Gen AI
- Data Privacy and Security
- Sensitive Data Handling: Automating data engineering tasks involves processing large volumes of potentially sensitive data. Ensuring data privacy and security is paramount to prevent data breaches and comply with regulations.
- Compliance with Regulations: Organizations must navigate complex regulatory environments, ensuring that automated processes comply with data protection laws such as GDPR and CCPA.
- Ethical Considerations
- Bias and Fairness: Gen AI models can inadvertently perpetuate biases present in the training data. Ensuring fairness and transparency in AI-generated outcomes is a significant challenge.
- Accountability: Determining accountability for decisions made by AI systems can be complex. Clear guidelines and frameworks are needed to address ethical concerns.
- Technical Challenges
- Data Quality Issues: Automating data engineering tasks requires high-quality input data. Poor data quality can lead to inaccurate AI models and erroneous outputs.
- Complexity of Implementation: Integrating Gen AI into existing data engineering workflows can be technically challenging. It requires expertise in both data engineering and AI technologies.
- Skill Gap
- Training and Upskilling: The integration of Gen AI in data engineering necessitates a skilled workforce capable of understanding and managing AI-driven processes. Organizations need to invest in training and upskilling their data engineers.
- Interdisciplinary Expertise: Effective implementation of Gen AI requires expertise in data engineering, AI, and domain-specific knowledge. Bridging these interdisciplinary gaps can be challenging.
- Cost of Implementation
- Initial Investment: The deployment of Gen AI technologies involves significant initial investment in terms of infrastructure, software, and training.
- Ongoing Maintenance: Maintaining and updating AI-driven systems require continuous investment in terms of resources and expertise.
The future of data engineering in digital product engineering lies in the innovative capabilities of Generative AI. By automating data processes, enhancing data modeling, and enabling real-time data processing, Gen AI is set to transform how digital products are developed and operated. As organizations navigate this new landscape, they must address challenges related to data privacy, ethical AI, and skill gaps to fully harness the potential of Gen AI. The synergy between data engineering and Gen AI promises to drive the next wave of digital innovation, creating smarter, more responsive, and personalized digital products.