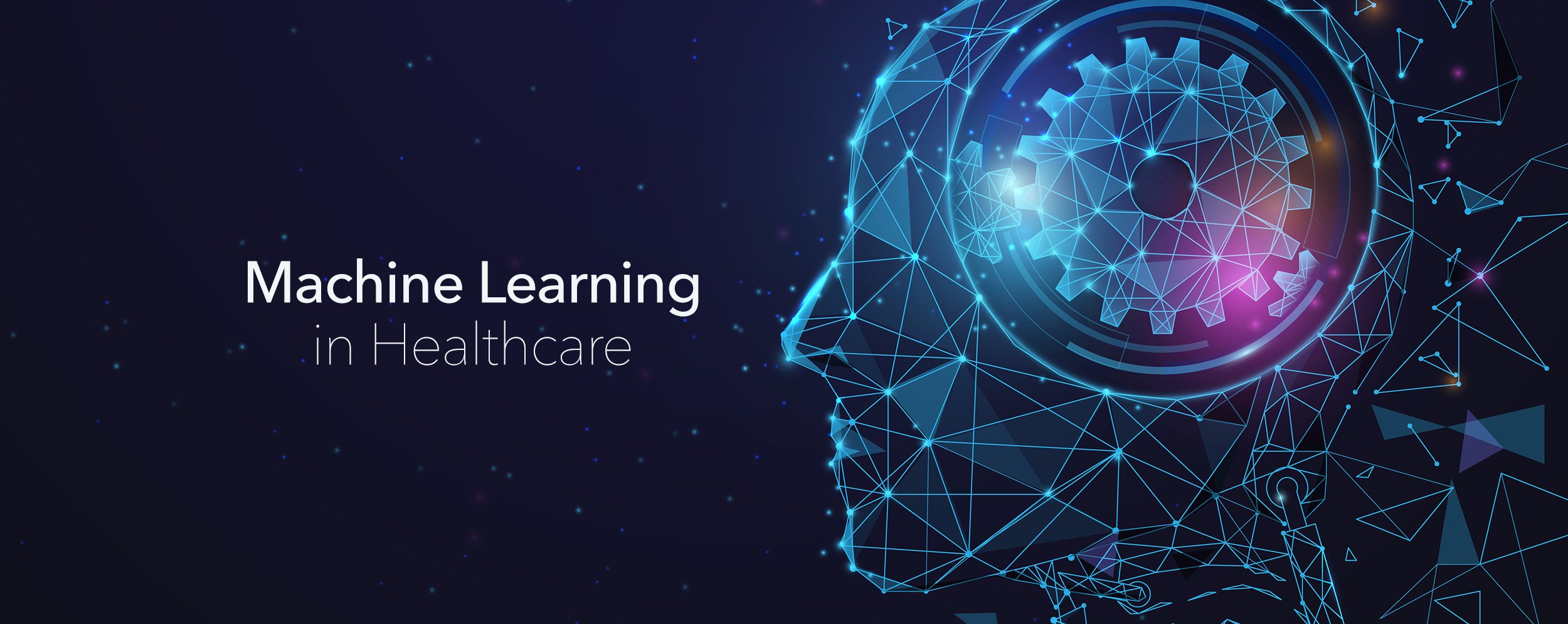
The advent of Artificial Intelligence (AI) has revolutionized numerous industries, and healthcare is no exception. Among the various applications of AI in healthcare, early disease detection is one of the most promising and impactful. Early detection of diseases can lead to timely intervention, improved patient outcomes, and reduced healthcare costs. This blog explores the role of AI in early disease detection, highlighting its potential, the challenges it faces, and the future of AI-driven diagnostics in the medical field.
A. Introduction to AI in Healthcare
Artificial Intelligence, at its core, refers to the ability of machines to mimic human intelligence, performing tasks such as learning, reasoning, problem-solving, and decision-making. In healthcare, AI systems are designed to analyze complex medical data, identify patterns, and make predictions that can assist healthcare professionals in diagnosing and treating diseases.
AI’s application in healthcare has grown rapidly in recent years, driven by advances in machine learning, deep learning, natural language processing, and data analytics. These technologies enable AI systems to process vast amounts of data, including medical records, imaging studies, genetic information, and clinical trial results, to generate previously unattainable insights.
B. The Importance of Early Disease Detection
Early detection of diseases is a critical aspect of healthcare that can significantly influence patient outcomes. Detecting a disease in its early stages often allows for more effective treatment, reduces the risk of complications, and increases the likelihood of a full recovery. This is particularly important for chronic and life-threatening conditions such as cancer, cardiovascular diseases, diabetes, and neurological disorders.
However, early detection can be challenging due to early symptoms’ subtle and often non-specific nature. Traditional diagnostic methods, such as imaging, blood tests, and physical examinations, may only sometimes be sensitive enough to detect diseases early. This is where AI comes into play, potentially enhancing the accuracy and speed of early disease detection.
AI Techniques in Early Disease Detection
Several AI techniques are being employed to improve early disease detection. These techniques leverage advanced algorithms and models to analyze medical data and identify early signs of disease. Some of the key AI techniques include:
A. Machine Learning (ML)
Machine learning is a subset of AI that focuses on developing algorithms that can learn from data and improve performance over time. In the context of early disease detection, ML algorithms are trained on large datasets containing medical records, imaging studies, and other health-related information. These algorithms can identify patterns and correlations that may indicate the presence of a disease even before symptoms become apparent.
For example, ML algorithms have been used to detect early signs of diabetic retinopathy, which can lead to blindness if left untreated. By analyzing retinal images, these algorithms can identify subtle changes in blood vessels and other structures that may indicate the onset of the disease.
B. Deep Learning (DL)
Deep learning is a more advanced form of machine learning that involves the use of artificial neural networks with multiple layers. These networks can process large amounts of data and identify complex patterns that may not be apparent to human observers.
In early disease detection, deep learning models have successfully analyzed medical images, such as X-rays, CT scans, and MRIs. For instance, deep learning algorithms have been developed to detect early-stage lung cancer from chest CT scans. These algorithms can identify small nodules that may indicate cancer, allowing for earlier diagnosis and treatment.
C. Natural Language Processing (NLP)
Natural language processing is a branch of AI that focuses on the interaction between computers and human language. NLP techniques analyze unstructured text data, such as medical records, clinical notes, and research articles.
In early disease detection, NLP can extract relevant information from electronic health records (EHRs) and identify patients who may be at risk of developing a particular disease. For example, NLP algorithms can scan clinical notes for mentions of symptoms, risk factors, or family history that may indicate the need for further screening or diagnostic tests.
D. Predictive Analytics
Predictive analytics involves using statistical models and AI algorithms to predict the likelihood of future events based on historical data. In healthcare, predictive analytics can identify individuals who are at high risk of developing certain diseases, allowing for targeted interventions and preventive measures.
For example, predictive analytics models have been developed to assess the risk of cardiovascular disease based on factors such as age, gender, blood pressure, cholesterol levels, and lifestyle choices. These models help healthcare providers identify patients who may benefit from lifestyle changes or medication to reduce risk.
E. Genomic Analysis
AI is also playing a significant role in analyzing genomic data for early disease detection. Genomic analysis involves examining an individual’s DNA to identify genetic mutations or variations that may increase the risk of certain diseases.
AI algorithms can analyze vast amounts of genomic data to identify patterns associated with specific diseases. For example, AI has been used to identify genetic markers associated with an increased risk of breast cancer. By analyzing a patient’s genetic profile, healthcare providers can recommend preventive measures or more frequent screenings to detect the disease at an early stage.
Applications of AI in Early Disease Detection
AI is being applied across various medical fields to improve the early detection of diseases. Some of the notable applications include:
A. Cancer Detection
Cancer is one of the leading causes of death worldwide, and early detection is critical for improving survival rates. AI has shown great promise in detecting various types of cancer at an early stage, including breast cancer, lung cancer, and colorectal cancer.
In breast cancer detection, AI algorithms have been developed to analyze mammograms and identify suspicious areas that may indicate the presence of tumors. These algorithms can detect cancerous lesions with high accuracy, even in cases where the tumors are small and not easily visible to radiologists.
Similarly, AI has been used to analyze colonoscopy images for the early detection of colorectal cancer. By identifying polyps and other abnormal growths, AI can assist in the early diagnosis and removal of precancerous lesions.
B. Cardiovascular Disease Detection
Cardiovascular diseases (CVDs) are a leading cause of death globally, and early detection is essential for preventing heart attacks, strokes, and other complications. AI is used to analyze various data, including electrocardiograms (ECGs), medical imaging, and patient records, to detect early signs of CVD.
For example, AI algorithms have been developed to analyze ECG data and detect irregular heart rhythms, such as atrial fibrillation, which can increase the risk of stroke. These algorithms can identify subtle changes in heart activity that may indicate the onset of a cardiovascular event, allowing for early intervention.
In addition, AI is being used to analyze imaging studies, such as coronary angiograms and cardiac MRIs, to detect blockages or abnormalities in the coronary arteries. Early detection of these issues can lead to timely treatment and reduced risk of heart attacks.
C. Neurological Disease Detection
Neurological diseases, such as Alzheimer’s disease, Parkinson’s disease, and multiple sclerosis, are often challenging to diagnose in their early stages. AI is helping to improve the early detection of these conditions by analyzing brain imaging, genetic data, and patient records.
For example, AI algorithms have been developed to analyze brain scans for early signs of Alzheimer’s disease. These algorithms can identify changes in brain structure, such as the accumulation of amyloid plaques, that may indicate the onset of the disease years before symptoms appear.
AI is also used to analyze speech patterns and motor function in patients with Parkinson’s disease. By detecting subtle changes in speech or movement, AI can assist in the early diagnosis of the disease and the initiation of treatment.
D. Infectious Disease Detection
The COVID-19 pandemic has highlighted the importance of early detection in controlling the spread of infectious diseases. AI has played a crucial role in the early detection of COVID-19 by analyzing medical imaging, such as chest X-rays and CT scans, and identifying patterns associated with the virus.
AI has also been used to develop predictive models that identify individuals at high risk of contracting infectious diseases based on travel history, contact tracing, and symptoms. These models can help public health authorities prioritize testing and containment efforts.
Beyond COVID-19, AI is being applied to the early detection of other infectious diseases, such as tuberculosis and influenza. By analyzing data from various sources, including medical records, laboratory results, and social media, AI can identify outbreaks and predict their spread, enabling timely intervention.
E. Diabetes Detection
Diabetes is a chronic condition that can lead to serious complications if not detected and managed early. AI improves the early detection of diabetes and its associated complications by analyzing patient data, such as blood glucose levels, lifestyle factors, and genetic information.
For example, AI algorithms have been developed to predict the risk of developing type 2 diabetes based on factors such as age, weight, family history, and lifestyle choices. These algorithms can identify individuals who are at high risk and recommend preventive measures, such as dietary changes or increased physical activity.
AI also monitors blood glucose levels in real-time and detects early signs of diabetic complications, such as diabetic retinopathy and neuropathy. By providing continuous monitoring and alerts, AI can help patients manage their condition more effectively and reduce the risk of complications.
Challenges and Ethical Considerations
While AI has the potential to revolutionize early disease detection, it also faces several challenges and ethical considerations that must be addressed.
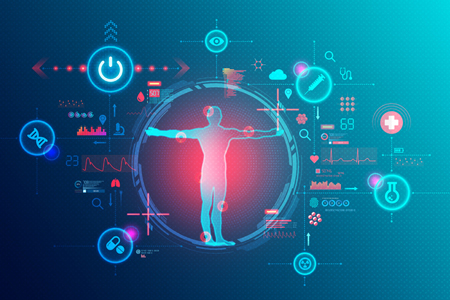
A. Data Privacy and Security
The use of AI in healthcare involves the collection and analysis of vast amounts of personal and medical data. Ensuring the privacy and security of this data is critical to maintaining patient trust and complying with regulations such as the Health Insurance Portability and Accountability Act (HIPAA) in the United States and the General Data Protection Regulation (GDPR) in Europe.
Healthcare providers and AI developers must implement robust data security measures to protect patient information from unauthorized access, breaches, and misuse. This includes encryption, secure data storage, and access controls.
B. Bias and Fairness
AI algorithms are only as good as the data on which they are trained. If the training data is biased or unrepresentative, the AI system may produce biased results, leading to disparities in healthcare outcomes. For example, if an AI model is trained primarily on data from one demographic group, it may not perform as well for patients from other groups.
To address this issue, it is essential to ensure that AI models are trained on diverse and representative datasets. Additionally, ongoing monitoring and evaluation of AI systems are necessary to identify and mitigate potential biases.
C. Interpretability and Transparency
AI algorithms, particularly deep learning models, can be complex and difficult to interpret. AI’s “black box” nature can make it challenging for healthcare providers to understand how the AI system arrived at a particular diagnosis or recommendation.
To build trust in AI systems, it is important to develop methods for explaining AI decisions in a way that clinicians and patients can understand. This includes clarifying the factors influencing the AI’s decision and allowing for human oversight and validation.
D. Regulatory and Legal Challenges
The use of AI in healthcare is subject to regulatory oversight to ensure patient safety and the efficacy of AI-driven diagnostics. However, existing regulatory frameworks may need to be fully equipped to address the unique challenges posed by AI.
Regulatory agencies, such as the U.S. Food and Drug Administration (FDA), are developing guidelines and standards for the use of AI in healthcare. These guidelines must address issues such as the validation of AI algorithms, the transparency of AI systems, and the accountability of healthcare providers using AI.
The Future of AI in Early Disease Detection
The future of AI in early disease detection is promising, with ongoing research and development expected to lead to even more advanced and accurate AI-driven diagnostic tools. Several key trends are likely to shape the future of AI in this field:
A. Integration with Wearable Devices
Wearable devices, such as smartwatches and fitness trackers, are becoming increasingly popular and can continuously monitor vital signs, activity levels, and other health metrics. AI algorithms can analyze the data collected by these devices to detect early signs of disease, such as irregular heart rhythms, changes in sleep patterns, or fluctuations in blood glucose levels.
As wearable technology advances, integrating AI with these devices will enable more personalized and proactive healthcare, allowing for early intervention and better disease management.
B. AI-Driven Biomarker Discovery
Biomarkers are measurable indicators of biological processes or conditions crucial in early disease detection. AI analyzes large datasets, such as genomic, proteomic, and metabolomic data, to identify new biomarkers associated with specific diseases.
The discovery of novel biomarkers can lead to the development of more accurate diagnostic tests and targeted therapies, improving the early detection and treatment of diseases such as cancer, Alzheimer’s disease, and autoimmune disorders.
C. AI in Telemedicine
Telemedicine has gained significant traction recently, particularly during the COVID-19 pandemic. AI has the potential to enhance telemedicine by providing remote diagnostic capabilities, enabling healthcare providers to detect diseases early and provide timely care to patients, regardless of their location.
For example, AI-powered diagnostic tools can be integrated into telemedicine platforms to analyze patient data, such as medical history, symptoms, and imaging studies, and provide real-time diagnostic support to healthcare providers during virtual consultations.
D. Collaborative AI and Human-AI Partnerships
The future of AI in early disease detection will likely involve greater collaboration between AI systems and healthcare professionals. Rather than replacing human clinicians, AI will be a valuable tool that enhances their ability to detect diseases early and make informed decisions.
Human-AI partnerships will involve integrating AI into clinical workflows. AI will provide diagnostic support while clinicians exercise their judgment and expertise to interpret the AI’s findings and determine the appropriate course of action.
E. Continuous Learning and Adaptation
AI systems can continuously learn and adapt as new data becomes available. This continuous learning capability will enable AI to stay up-to-date with the latest medical research, treatment guidelines, and emerging diseases, ensuring that AI-driven diagnostics remain accurate and relevant.
As AI systems evolve, they will become even more effective at detecting diseases early and improving patient outcomes.
Artificial Intelligence is increasingly important in early disease detection, potentially revolutionizing healthcare by enabling more accurate, timely, and personalized diagnostics. Through advanced AI techniques such as machine learning, deep learning, natural language processing, and predictive analytics, AI is helping healthcare providers detect diseases at their earliest stages when treatment is most effective.
While adopting AI in early disease detection presents challenges, including data privacy, bias, and regulatory considerations, the benefits of AI-driven diagnostics are undeniable. As AI technology advances, it will be critical in improving patient outcomes, reducing healthcare costs, and ultimately saving lives.
The future of early disease detection lies in the continued integration of AI into healthcare, focusing on collaboration between AI systems and healthcare professionals. By harnessing the power of AI, we can move closer to a future where early detection is the norm rather than the exception, leading to better health outcomes for individuals and populations worldwide.