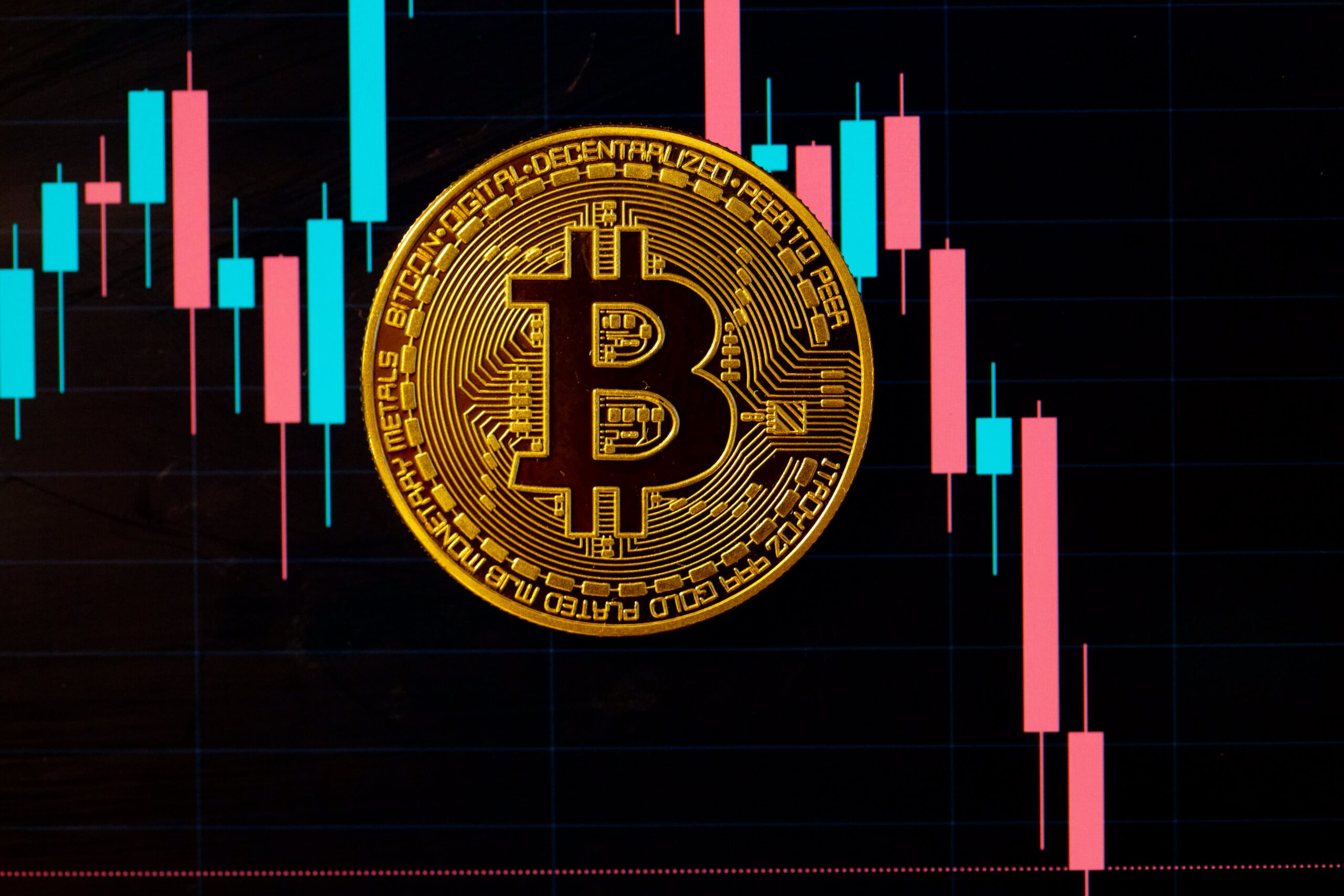
Bitcoin, the pioneer of cryptocurrencies, has garnered significant attention due to its volatile price movements and potential for high returns. Predicting its price accurately is a challenge that has intrigued both investors and researchers. One innovative approach gaining traction in this domain is the use of neuro-fuzzy techniques. This blog explores how these advanced methods can be applied to forecast Bitcoin prices effectively.
Understanding Bitcoin Price Volatility
Bitcoin’s price is influenced by a multitude of factors, including market demand, regulatory news, technological advancements, and macroeconomic trends. The complexity and interdependence of these variables make accurate prediction a daunting task. Traditional statistical methods often fall short due to the non-linear and dynamic nature of Bitcoin’s price movements.
What are Neuro-Fuzzy Techniques?
Neuro-fuzzy techniques combine the learning capabilities of neural networks with the human-like reasoning style of fuzzy logic. This hybrid approach leverages the strengths of both methodologies to model complex systems with greater accuracy.
- Neural Networks: These are computational models inspired by the human brain, capable of identifying patterns and learning from data. They excel in handling large datasets and uncovering intricate relationships among variables.
- Fuzzy Logic: This is a form of many-valued logic that deals with reasoning that is approximate rather than fixed and exact. In the context of Bitcoin price prediction, fuzzy logic helps in dealing with uncertainties and imprecise information, which are inherent in financial markets.
Why Use Neuro-Fuzzy Techniques for Bitcoin Forecasting?
- Handling Non-Linearity: Bitcoin’s price movements are highly non-linear. Neuro-fuzzy systems can capture these non-linear relationships more effectively than traditional linear models.
- Robustness to Noise: Financial data, including Bitcoin prices, are often noisy. Neuro-fuzzy techniques are robust to such noise, providing more reliable predictions.
- Adaptive Learning: As market conditions change, neuro-fuzzy models can adapt and update their rules and parameters, ensuring that the predictions remain relevant.
- Human-Like Reasoning: By incorporating fuzzy logic, these models can mimic human reasoning, making sense of imprecise and ambiguous information which is common in financial markets.
Implementing Neuro-Fuzzy Techniques for Bitcoin Price Prediction
Step 1: Data Collection
Gather historical Bitcoin price data along with relevant market indicators. This could include volume, market sentiment, macroeconomic variables, and more.
Step 2: Data Preprocessing
Clean the data to handle missing values, outliers, and noise. Normalize the data to ensure that it is suitable for input into the neuro-fuzzy model.
Step 3: Feature Selection
Identify the most relevant features that influence Bitcoin prices. This step is crucial as it impacts the model’s accuracy and efficiency.
Step 4: Model Building
Develop a neuro-fuzzy model using frameworks like ANFIS (Adaptive Neuro-Fuzzy Inference System). Train the model on historical data to learn the underlying patterns and relationships.
Step 5: Model Validation
Validate the model using a separate dataset to ensure its accuracy. Techniques like cross-validation can help in assessing the model’s performance.
Step 6: Prediction
Use the trained and validated model to predict future Bitcoin prices. Continuously monitor the model’s performance and update it as necessary to adapt to changing market conditions.
Bitcoin price forecasting remains a challenging yet fascinating task. Neuro-fuzzy techniques offer a promising solution by combining the adaptive learning of neural networks with the approximate reasoning of fuzzy logic. By leveraging these advanced methods, investors and researchers can gain better insights into Bitcoin’s price movements, enabling more informed decision-making.
As the cryptocurrency market continues to evolve, the application of neuro-fuzzy techniques will likely become more prevalent, paving the way for more accurate and reliable financial forecasting.