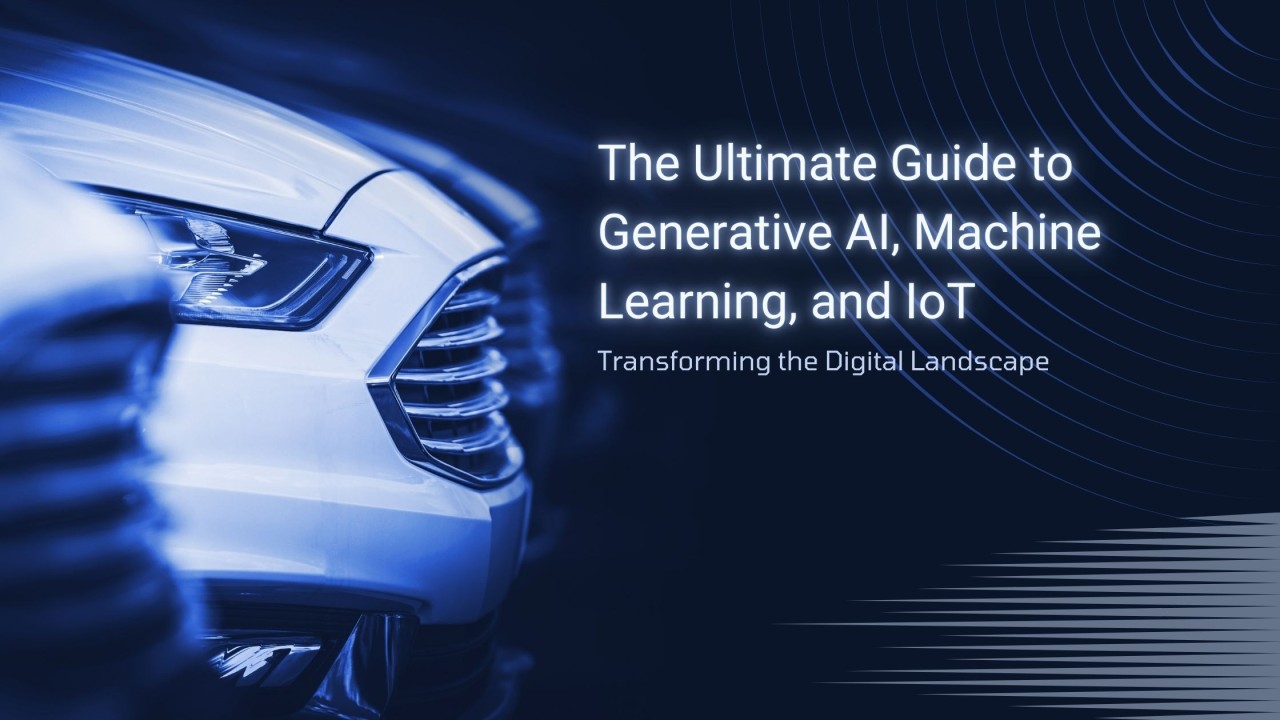
Top 10 Ultimate Guide to Generative AI
The ultimate guide to Generative AI has revolutionized the way we interact with technology, enabling machines to create text, images, music, and even entire virtual environments. It’s a rapidly evolving field that combines deep learning models, massive datasets, and advanced algorithms to produce creative outputs that mimic human-like capabilities. Whether you’re a business professional, a developer, or an AI enthusiast, understanding the core concepts and applications of generative AI is essential. Here’s an in-depth look at the top ten ultimate guide to generative AI to give you a comprehensive understanding of this ground-breaking technology.
1. What Is Generative AI?
Generative AI refers to algorithms or models that can generate new content, whether it be images, text, audio, or even video, from scratch or based on given inputs. Unlike traditional AI models, which are designed primarily for recognizing patterns and making predictions based on historical data, generative AI goes a step further by producing original content. It uses machine learning models such as GANs (Generative Adversarial Networks), VAEs (Variational Autoencoders), and autoregressive models, which help generate content that closely mirrors the patterns of the data on which they have been trained.
Generative AI models create data that did not exist before, based on the underlying patterns and features learned from a dataset. For example, a generative AI model trained on a large dataset of human faces can generate entirely new images of faces that are not based on any real individual. This ability makes generative AI extremely powerful in areas like content creation, synthetic media, and data augmentation.
2. How Does Generative AI Work?
Generative AI relies on advanced machine learning techniques. Two of the most prominent methods are:
- Generative Adversarial Networks (GANs): GANs involve two neural networks—a generator and a discriminator—working together. The generator creates new data, while the discriminator evaluates its authenticity. This feedback loop improves the quality of the generated content over time.
- Transformer Models: These are particularly effective for natural language processing tasks. Models like GPT (Generative Pre-trained Transformer) use vast amounts of text data to learn language patterns and generate coherent responses.
The effectiveness of generative AI depends on the quality and diversity of training data, as well as the sophistication of the model architecture.
3. Applications of Generative AI in Various Industries
Generative AI has a broad range of applications across various fields, revolutionizing industries and enabling new opportunities for creativity, automation, and innovation. Below are some of the key areas where generative AI is making a significant impact:
- Content Creation
One of the most exciting uses of generative AI is in content creation. Writers, marketers, and content creators use AI-driven tools to generate articles, blogs, social media posts, and more. For instance, GPT models are now widely used to generate human-like text, enabling businesses to scale their content creation efforts. With the help of AI, even complex pieces such as research papers or technical documents can be generated efficiently. - Image and Video Generation
Generative AI models like GANs are transforming how we create images and videos. These models can generate hyper-realistic images, art, and even short video clips. Artists and designers are leveraging AI to explore new creative possibilities, while marketers and advertisers use AI to produce customized visuals for campaigns. - Music Composition
Generative AI is also revolutionizing the music industry. AI models can compose music in different styles, genres, and tempos. Platforms like Open Ai’s Muse Net and Juke deck are using AI to generate original music that can be used in advertisements, films, games, or personal projects. Musicians also use AI tools to explore new compositions or enhance their existing work. - Customer Service and Chatbots
AI-powered chatbots are widely used for customer support across industries. These systems leverage natural language processing (NLP) and generative AI to offer personalized responses to customer queries. With generative AI, these bots can handle more complex inquiries and provide more human-like interactions, improving the overall customer experience.
4. Benefits of Generative AI
The adoption of generative AI offers several advantages to businesses and individuals, from enhancing creativity to driving efficiency. Below are some key benefits:
- Enhanced Creativity and Innovation
Generative AI empowers creators by providing them with new tools to explore their ideas. Artists, writers, and musicians can experiment with AI to generate unique content, discover new patterns, and push the boundaries of their creativity. - Cost and Time Efficiency
Generating content manually can be time-consuming and expensive. With generative AI, businesses can automate much of the content creation process, significantly reducing both time and costs. This is especially beneficial in industries like marketing, where content needs to be produced at scale. - Personalization at Scale
Generative AI models can help businesses offer highly personalized experiences to their customers. Whether it’s generating tailored recommendations, creating personalized ads, or developing unique content for individual users, generative AI allows businesses to provide more relevant and targeted services.
5. Ethical Considerations in Generative AI
While generative AI is powerful, it also raises ethical concerns:
- Bias in AI Outputs: Models can replicate biases present in training data.
- Deepfakes: The ability to create realistic fake videos poses security and privacy threats.
- Copyright Issues: AI-generated content often uses existing works as a basis, leading to questions about intellectual property.
Addressing these concerns requires a combination of regulatory frameworks, transparent development practices, and public awareness.
6. The Role of Generative AI in Business
Businesses are leveraging generative AI to enhance productivity and customer engagement:
- Marketing: Personalized campaigns powered by AI drive higher engagement rates.
- Product Development: AI-generated prototypes and simulations speed up the innovation process.
- Customer Support: AI chatbots provide instant, tailored responses to customer queries.
Generative AI enables organizations to optimize operations, improve customer experiences, and maintain a competitive edge.
7. Tools and Platforms for Generative AI
A wide array of tools makes generative AI accessible to developers and businesses:
- Open AI GPT Models: Used for text generation and conversational AI.
- DALL·E and Mid Journey: Create visually stunning images from text prompts.
- Runway ML: Offers tools for video editing and creative applications.
These platforms provide user-friendly interfaces, making it easier for non-technical users to harness the power of generative AI.
8. Challenges and Limitations of Generative AI
Despite its potential, generative AI also presents certain challenges that need to be addressed:
- Ethical Concerns
One of the biggest challenges associated with generative AI is the potential misuse of the technology. AI-generated deepfakes, for example, can be used to spread misinformation or impersonate individuals. There is also concern about AI creating biased or harmful content based on the data it was trained on. - Quality Control and Accuracy
While generative AI models can produce impressive content, they are not perfect. In some cases, the generated content may contain errors, inaccuracies, or inappropriate elements. Ensuring that AI-generated content is of high quality and free from errors is a significant challenge. - Data Privacy Issues
Generative AI requires vast amounts of data to train effectively. However, there are concerns about data privacy, especially when using personal or sensitive data to train models. Safeguarding user data and ensuring compliance with privacy regulations is crucial for responsible AI development.
9. The Future of Generative AI
The future of generative AI is bright, with significant advancements expected in the coming years. As AI models become more sophisticated, they will be able to generate even more realistic and high-quality content. The following trends are likely to shape the future of generative AI:
- Improved AI Models
With advancements in machine learning and neural networks, generative AI models will become increasingly accurate and efficient. We can expect AI to generate content that is indistinguishable from human-produced work. - Greater Integration with Industries
Generative AI will continue to be integrated into a variety of industries, including healthcare, entertainment, education, and finance. As the technology matures, we will see more practical applications and innovative use cases. - Ethical AI Development
The ethical implications of generative AI will become more prominent, and efforts to regulate AI will increase. As AI systems become more powerful, it is essential to ensure that they are developed and used in ways that benefit society as a whole.
10. How to Get Started with Generative AI
If you’re interested in exploring generative AI, here’s how to begin:
- Learn the Basics: Understand foundational concepts like machine learning and deep learning.
- Experiment with Tools: Start with user-friendly platforms like ChatGPT or DALL·E.
- Take Courses: Platforms like Coursera and Udemy offer specialized courses on generative AI.
- Collaborate with Experts: Partner with AI professionals to implement generative AI in your business.
By taking a step-by-step approach, you can harness the potential of generative AI to achieve your goals.
Key Types of Generative AI Models
There are several types of generative AI models, each with distinct characteristics and applications. Here are the most common ones:
- Generative Adversarial Networks (GANs)
GANs consist of two neural networks—a generator and a discriminator—that work together. The generator creates data (images, text, etc.), while the discriminator evaluates the generated data’s quality by comparing it to real data. This process leads to the generator producing increasingly realistic content over time. GANs are widely used in image generation, video creation, and even in enhancing video games and movies. - Variational Autoencoders (VAEs)
VAEs are used for generating new data by learning the underlying distribution of the input data. They are particularly effective in areas like image compression and data augmentation. VAEs are often used when it is necessary to generate content that is diverse and exhibits high variability. - Autoregressive Models
Autoregressive models, such as GPT (Generative Pre-trained Transformer), are used to generate sequences of data, primarily in the realm of text generation. These models predict the next element in a sequence based on the previous ones. GPT-3 and GPT-4, for example, are capable of generating human-like text, making them ideal for applications like chatbots, content creation, and even code generation.
Conclusion
The ultimate guide to Generative AI is a transformative technology that is poised to revolutionize industries and reshape the creative landscape. From content generation to healthcare innovations, its applications are vast and varied. However, with great power comes great responsibility. It is essential for developers, businesses, and policymakers to ensure that the ultimate guide to generative AI is used ethically and responsibly. As the technology continues to evolve, we can expect even more exciting possibilities for the future of AI-driven content and innovation.
By understanding the principles, benefits, challenges, and future potential of ultimate guide to generative AI, businesses and individuals can harness its power to stay ahead in an increasingly AI-driven world. By leveraging this ultimate guide to generative ai ultimate guide to generative ai
.